Conference Program
Chair: Leslie Anne Fendt, Roche, Switzerland
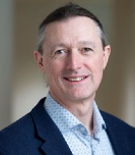
Roche Information Solutions, Switzerland
- driving efficiency and productivity in healthcare systems
- integrating and activating unstructured and disparate data
- enhancing insights and patient outcomes
- empowering patients, carers and healthcare professionals
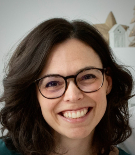
Dalle Molle Institute for Artificial Intelligence Studies (IDSIA USI-SUPSI), Switzerland & Swiss Institute of Bioinformatics (SIB) Switzerland
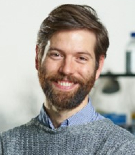
University Hospital Zürich, Switzerland
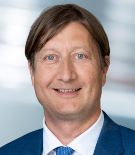
University of Zurich (UZH), Switzerland
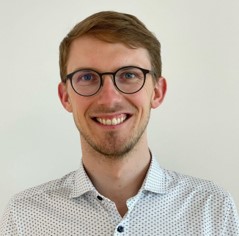
Roche, Switzerland
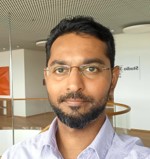
Roche, Switzerland
The manufacturing of large molecule drug substances is inherently complex, resulting in significant variability in the Active Pharmaceutical Ingredients (API) produced. Often, the causes of this variability are unknown, posing challenges to reproducibility, supply chain management, raw material consumption, equipment usage, and planning overheads. To address these issues, AI and machine learning (ML) are employed to gain insights into variability and mitigate it, aiming to produce optimal “golden batches.” Increased API production (measured in kilograms) enhances business value by lowering the Cost of Goods Sold (COGS), conserving raw materials, streamlining the supply chain, reducing dependence on external manufacturing, and enabling quicker root cause analysis during manufacturing deviations. Additionally, AI/ML applications provide shop floor personnel with guided recommendations to ensure optimal decision-making for achieving golden batches.
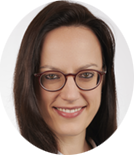
University of Applied Sciences and Arts Northwestern Switzerland (FHNW), Swiss Institute of Bioinformatics and aiNET GmbH, Switzerland
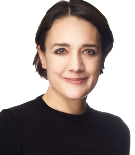
Roche, Switzerland
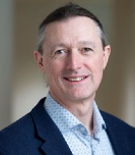
Roche Information Solutions, Switzerland
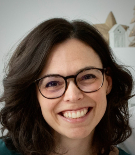
Dalle Molle Institute for Artificial Intelligence Studies (IDSIA USI-SUPSI), Switzerland & Swiss Institute of Bioinformatics (SIB) Switzerland
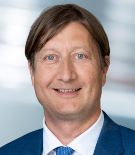
University of Zurich (UZH), Switzerland
Moderator: Enkelejda Miho, Switzerland
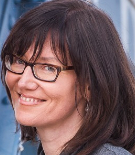
Roche Innovation Center Zurich, Switzerland
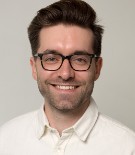
University of Oslo, Norway
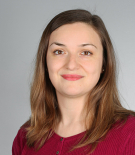
University of Basel, Switzerland & Swiss Institute of Bioinformatics (SIB) Switzerland
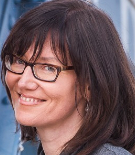
Roche Innovation Center Zurich, Switzerland
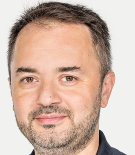
University of Applied Sciences and Arts Northwestern Switzerland (FHNW) & Swiss Institute of Bioinformatics (SIB), Switzerland
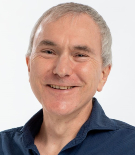
Molecular Partners, Switzerland
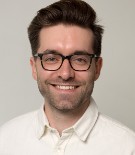
University of Oslo, Norway
Moderator: Leila T. Alexander, Switzerland
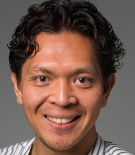
Novo Nordisk, Denmark
The recent Nobel Prize in Chemistry highlighted the impact of artificial intelligence (AI) in protein design. Yet, antibody design, a subset of the protein design, remains a large challenge. The success rate of AI namely AlphaFold-Multimer version 2 (AF2) for antibody-antigen complexes has been benchmarked at 20%-30%, AlphaFold version 3 (AF3) improved the success rate to 40%. In contrast, the success rates for protein-protein complexes peak at 75% for AF2 and upward 80% for AF3. We discuss herein opportunities and challenges in advancing the frontier of protein design for the design of antibody therapeutics. We highlight the need for data in terms of scale and protein-protein interaction class (disordered vs ordered). The utility of deep learning in capturing the latent embedding space to describe long-range interaction, biophysical properties and downstream prediction task. Finally, the value in combining deep and shallow learning to operationalize AI for antibody design.
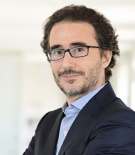
University of Basel, Switzerland
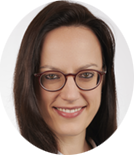
University of Applied Sciences and Arts Northwestern Switzerland (FHNW), Swiss Institute of Bioinformatics and aiNET GmbH, Switzerland
Carine Poussin, AI for Life Sciences, CSEM, Switzerland
Kyaw Zin Thann, Myanmar Country Program, PATH, Myanmar
Michele Svanera, Centre for Cognitive Neuroimaging, University of Glasgow, United Kingdom
Dalit Porat Ben Amy, Tzafon Medical Center, Bar Ilan University, CatAI – Oralyze, Israel
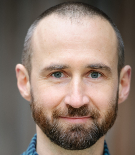
aboutDigitalHealth.com, Germany
In 1966, Joseph Weizenbaum, a computer scientist at MIT, developed ELIZA, one of the earliest chatbots. Despite its primitive technology, patients interacting with ELIZA believed they were talking to an empathetic machine. In reality, ELIZA merely mirrored statements in a manner akin to a psychotherapist, creating the illusion of conversation—a phenomenon later termed the “Eliza effect.” This effect highlights our tendency to anthropomorphize machines, attributing them human features such as intelligence and empathy.
Today, modern generative AI models like ChatGPT exhibit a more sophisticated version of the Eliza effect. Although these models do not “understand” language in the human sense, their ability to analyze and generate text with perfect grammar and context strengthens the illusion of intelligence. With advances in AI, we are now on the brink of interacting with personalized bots capable of booking appointments or even carrying out emotionally expressive conversations.
The question remains: how will this deepening human-machine interaction evolve? As AI increasingly resembles human communication, will we trust these systems as we do family, doctors, or advisors? How far will we extend our trust to machines, even in intimate or specialized roles, such as healthcare? Exploring these questions requires a deeper understanding of psychology and human behavior in response to technology.
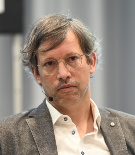
University of Basel, Switzerland
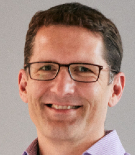
Novartis Foundation, Switzerland
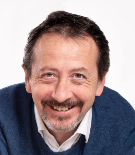
StratejAI, Italy
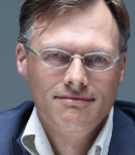
Swiss Made Software, Switzerland
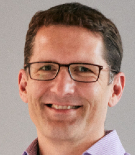
Novartis Foundation, Switzerland
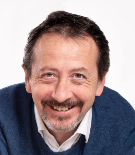
StratejAI, Italy
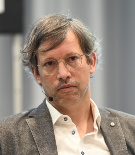
University of Basel, Switzerland
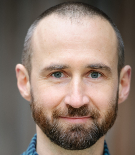
aboutDigitalHealth.com, Germany
Moderator: Thomas Brenzikofer, Switzerland
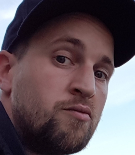
University of Basel, Switzerland & & Swiss Institute of Bioinformatics (SIB) Switzerland
This lecture explores the transformative potential of consistently embedding cutting-edge software technologies within an Open Science framework to maximize productivity and actionable outcomes for global health. It starts off by introducing some grand challenges of medical research in the 21st century and how Open Science proposes to address them. As an example, it then highlights how Open Standards facilitate seamless data sharing, collaboration, and reproducibility, breaking down traditional silos in medical research and shifting data analysis paradigms, as outlined in international initiatives such as the Federated European Genome-Phenome Archive (FEGA) and the European Genomic Data Infrastructure (GDI). Finally, it attempts to extrapolate from these developments a vision for an open, federated and standards-based software platform that connects researchers, healthcare practitioners, patients and AI to collectively champion global health challenges.